Loan data analysis involves scrutinizing various aspects of loans to derive meaningful insights that can guide decision-making, risk management, and strategic planning.
In this article, we will explore some of the best practices for analyzing loan data to ensure accuracy, reliability, and actionable results.
When dealing with loan data, especially in the context of 대출사이트, it is crucial to adopt a systematic and thorough approach.
Page Contents
Understand Your Data
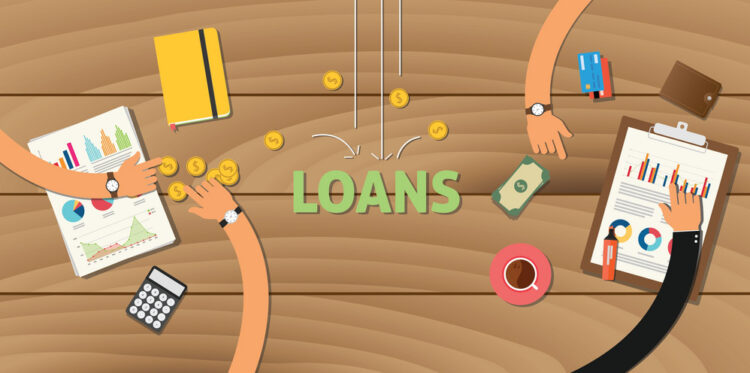
Source: vectorstock.com
Before diving into any analysis, it’s essential to have a deep understanding of the data at hand. This involves knowing the source of the data, the structure, and the variables included. Typically, loan data will encompass borrower details, loan amounts, interest rates, repayment terms, and payment histories. Ensuring the data is clean and free from errors or inconsistencies is a critical first step. This might involve checking for missing values, outliers, and ensuring all data entries are in a consistent format.
Define Clear Objectives
Having a clear objective is vital for effective loan data analysis. Whether you’re looking to assess the risk profile of borrowers, understand repayment behaviors, or identify trends in loan performance, defining your goals will guide your analytical approach.
Data Cleaning and Preparation
Data cleaning is a fundamental step in preparing your dataset for analysis. This involves handling missing data, removing duplicates, and correcting inconsistencies. Techniques such as imputation can be used to handle missing values, while normalization and standardization can help in dealing with variables measured on different scales. A clean dataset is crucial for reliable and valid analysis outcomes.
Exploratory Data Analysis (EDA)
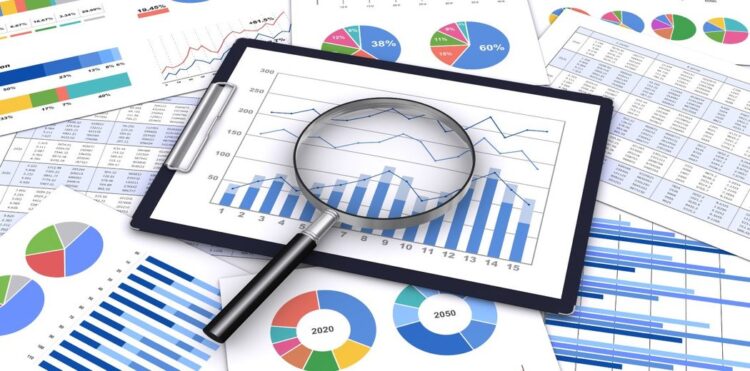
Source: micoope.com.gt
Exploratory Data Analysis (EDA) is a critical step that helps in understanding the underlying patterns and relationships within the data. During EDA, you can use statistical summaries and visualizations to identify trends, correlations, and anomalies. Tools such as histograms, scatter plots, and box plots are particularly useful in this phase.
Feature Engineering
Feature engineering involves creating new variables that can enhance the predictive power of your model. In the context of loan data, this might include calculating the debt-to-income ratio, creating binary indicators for late payments, or deriving the length of credit history. These new features can provide additional insights and improve the performance of your predictive models.
Choosing the Right Analytical Techniques
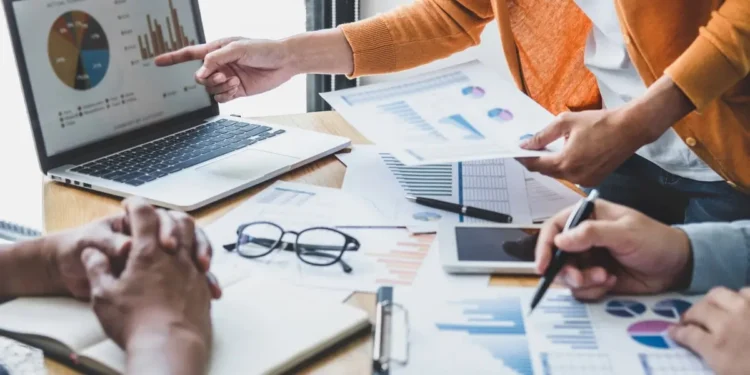
Source: careerfoundry.com
The choice of analytical techniques will depend on your objectives and the nature of your data. Common techniques include regression analysis, classification algorithms, and clustering. For instance, logistic regression can be used to predict the probability of loan default, while clustering can help segment borrowers into distinct groups based on their characteristics..
Conclusion
Analyzing loan data effectively requires a combination of thorough data understanding, clear objectives, meticulous data preparation, and the application of appropriate analytical techniques. By following these best practices, financial institutions and analysts can derive valuable insights that inform decision-making and contribute to improved loan management and risk mitigation. With the rapid advancements in technology, leveraging sophisticated tools can further enhance the accuracy and efficiency of loan data analysis.